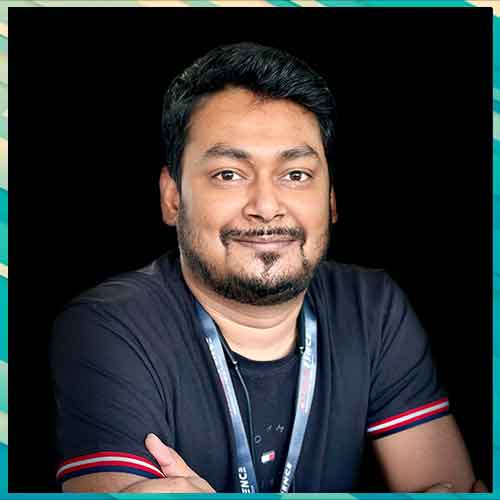
AttackFence is transforming cybersecurity with its cutting-edge Network Detection and Response (NDR) platform, which focuses on detecting sophisticated threats such as lateral movements, data exfiltration, and insider attacks. The platform utilizes behavioural analysis and flow metadata for real-time detection, minimizing reliance on traditional signatures or logs. In an insightful interaction with VARINDIA, Vivek Singh, Engineering Lead at AttackFence, shares how the NDR platform tackles challenges like encrypted traffic and AI integration, while also adapting to hybrid and multi-cloud environments to enhance threat detection and operational efficiency. Excerpts:
What are the main cybersecurity threats that NDR is designed to detect and respond to?
NDR focuses on detecting lateral movement, data exfiltration, command and control (C2) traffic, and insider threats — particularly those that evade traditional defenses like firewalls or endpoint protection. It helps organizations to detect even the stealthiest of attacks and APTs.
How does today's NDR differ from traditional network security tools like IDS, IPS, or SIEM?
Unlike IDS/IPS that rely on known signatures, or SIEMs that depend on logs, NDR uses flow metadata, behavioural analysis to detect anomalies and unknown threats in real-time. It adds a deep, contextual view to existing defenses.
What unique challenges does your NDR address that others in the market do not?
Our NDR is designed to work even in low-visibility or encrypted environments, uses a lightweight system for resource efficiency, and supports both edge and cloud deployments with a strong focus on behaviour-based detection models. Our multipronged analytics engine utilises a mesh of ML algorithms along with IOC and BIOC rules for creating anomaly and alerts.
How are AI and machine learning transforming NDR capabilities today?
AI enables adaptive baselining and faster detection of unknown threats. ML models help in prioritizing alerts based on risk, reducing noise and focusing analyst attention where it matters most.
In an increasingly cloud-native world, how does your NDR adapt to hybrid and multi-cloud environments?
Our platform supports cloud-native sensors that can capture traffic into flow logs forwarded from cloud providers, ensuring deep visibility without compromising the efficiency/throughput of source devices. We have benchmarked our solution to be successfully able to receive and process traffic forwarded by vTAP.
How important is encrypted traffic analysis in modern NDR, and how does your platform tackle it?
Very important. Over 90% of traffic is encrypted today. We focus on analyzing metadata, TLS handshake parameters, and behavioural patterns rather than breaking encryption, ensuring detection without violating privacy.
How do you balance privacy concerns while deeply analyzing network traffic?
We avoid payload inspection and instead rely on flow-level metadata and behavioural anomalies. Where DPI is needed, it’s policy-driven and scoped to ensure compliance with regulations.
What role do regulations, like GDPR, DPDPA and HIPAA, play in shaping how you design NDR solutions?
As we’ve already discussed, over 90% of network traffic today is encrypted. Since we neither decrypt nor inspect payloads, and our detection techniques rely solely on metadata, our solution is inherently designed to align with data protection regulations.
Can you share an example of how your NDR detected a sophisticated threat that traditional defenses missed?
During a POC, a customer flagged recurring IOC alerts across multiple systems. While their endpoint solution was blocking execution, there was a risk it could succeed on unmanaged devices or evolve to bypass defenses. Our NDR correlated file transfers with alert timestamps, revealing three pivoting machines spreading the payload. Isolating them stopped the organization-wide alerts, something traditional tools had missed. This was a classic case of lateral movement detection.
What’s the biggest ROI that enterprises can expect by adopting NDR?
The biggest ROI enterprises can expect is measurable risk reduction—achieved through faster threat detection, proactive threat hunting, quicker incident response, and fewer false positives. This directly leads to lower operational costs, minimized downtime, and stronger protection against reputational and financial damage.
What are the top five use cases for NDR?
The top five use cases are:
1. Holistic insight into activity across on-premises, cloud, and hybrid ecosystems
2. Lateral movement detection
3. Command and Control (C2) traffic detection
4. Encrypted Traffic Analysis (without decryption)
5. Data Exfiltration Detection
How do you see NDR evolving over the next 3-5 years? Will it merge with XDR, AI-driven security, or something bigger?
In my view, NDR is going to become far more autonomous and tightly integrated into the broader security ecosystem—especially with XDR and SOC automation. We’re already seeing early signs of AI playing a bigger role, and I think that trend will accelerate.
Over the next few years, I expect NDR to move beyond just detection and start making real-time decisions—leveraging unsupervised learning to spot unknown threats and even taking autonomous actions like isolating compromised systems. The ability to correlate network activity with endpoint and identity data will be key.
What advice would you give enterprises just starting to invest in NDR technology?
Start with visibility and understand your network baseline. Choose NDR solutions that integrate easily with your environment and offer flexible deployment models (on-prem, cloud, hybrid).
See What’s Next in Tech With the Fast Forward Newsletter
Tweets From @varindiamag
Nothing to see here - yet
When they Tweet, their Tweets will show up here.